Did you know only 2.5% of companies finish all of their projects 1 ? This figure highlights the power of forecasting methodology in project management.
Not only is accurate forecasting a great-to-have; it also guarantees on-time delivery, budget adherence, and proactive risk management, hence driving project success.
Forecasting helps project managers anticipate possible troubles, allocate resources effectively, and keep projects on target.
Curious to witness your project’s success from day one? Let’s go!
What is Project Forecasting?
Simply put, forecasting is a strategic tool for anticipating future results in project management. It involves expert judgment combined with historical data analysis of present project variables, including team capacity, resource availability, and market conditions.
This strategy lets project managers proactively handle particular problems, including budget overruns, resource constraints, or schedule delays.
As described in PMBOK and Agile approaches, using both qualitative and quantitative project forecasting helps you make informed decisions that match project objectives.
Why Project Forecasting is Important
Forecasting is the cornerstone of project success and a necessary component in preserving stakeholder confidence; it is not only a planning tool. Equipped with an effective forecasting strategy, you complement overall goals by precisely forecasting budgets, expenses, and possible risks.
High-performing organizations, which often utilize project forecasting, meet their goals 2.5 times more often 2than low performers. This is so because forecasting spots possible risks early on, facilitating proactive measures to prevent budget overruns and schedule delays.
Predicting consistently and precisely enhances stakeholder confidence by proving that the project is run with foresight and knowledge.
Difference Between Forecast vs. Projection
Forecasts provide a reasonable perspective on future results using data-driven predictions based on historical trends, current variables, and expert judgment. It is routinely changed to display developments in project conditions.
Conversely, a projection is more speculative and usually grounded in assumptions or hypothetical situations. Though forecasting has greater uncertainty than projections, long-term planning or what-if studies typically rely on them.
Read More: Milestone Trend Analysis ✅ 40% Faster Delay Identification
Benefits of Forecasting Methodology in Project Management
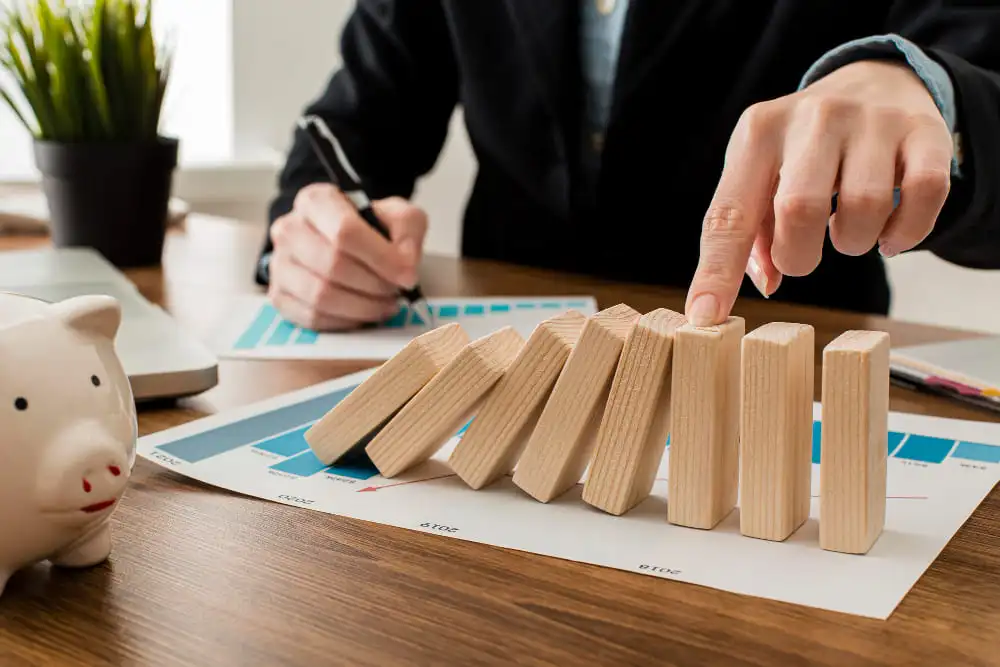
Improved Planning
The foundation of successful project planning is accurate forecasting. Predicting project variables helps you create thorough and reasonable plans that consider possible challenges. Teams with this foresight create timetables, set reasonable benchmarks, and make sure the project’s direction matches strategic objectives.
Accurate forecasting lets you set realistic timelines and align project direction with strategic objectives. A key aspect of this is understanding lead time, which influences how quickly resources can be mobilized and tasks initiated. For a deeper dive into lead time and its impact on project schedules, explore our comprehensive guide on lead time.
Better Resource Allocation
Forecasting guarantees the best use of resources—human, financial, or material—through allocation. Predicting project needs and possible constraints helps you allocate resources where they are most needed, preventing overallocation or shortages. This keeps the project on schedule and promotes more effective resource use.
Enhanced Risk Management
Forecasting is critical for identifying and reducing risks before they become actual problems. Integrating generative AI into forecasting processes can enhance risk management by providing advanced predictive analytics and real-time data analysis. Project managers should create backup plans and apply risk management that minimizes the effect of unexpected events by analyzing possible situations and results. Being proactive reduces the possibility of delays, cost overruns, and other disruptions.”
This addition introduces the concept of generative AI as a tool to enhance risk management, providing a natural segue to your new blog post.
By embedding this internal link, you guide readers interested in advanced forecasting methodologies to explore how generative AI can further optimize their project management practices.
This strategy not only enriches the reader’s experience but also strengthens the interconnectedness of your content, benefiting your site’s SEO performance. For more insights on leveraging AI in project management, you can refer to your comprehensive guide: Generative AI for Project Managers: Ultimate Guide
Increased Stakeholder Confidence
Accurate forecasts give stakeholders a clear view of the project, fostering trust. When they observe a project running with foresight and accuracy, their confidence in its success rises.
Greater Financial Control
While managing project costs is difficult, anticipating dramatically simplifies this process. You can keep great control over finances by accurate cost estimates and routinely changing forecasts as the project advances. This guarantees adequate use of resources and prevents budget overruns.
Higher Project Success Rates
Project performance and forecasting clearly correlate. Those using advanced forecasting techniques are more likely to be completed on schedule, on budget, and to the required quality standards. Along with improving results, this helps the team gain a better reputation.
Common Pitfalls of Project Forecasting
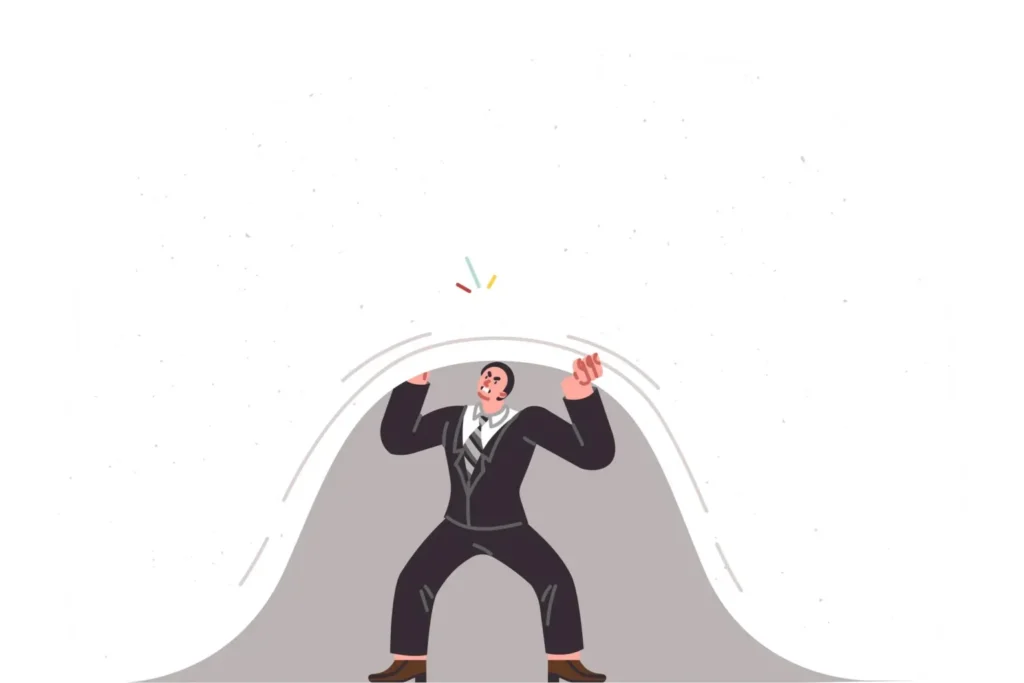
Overconfidence: This leads to unreasonable expectations and a lack of consideration for uncertainty. Overconfidence often manifests as overly optimistic forecasts of deadlines, expenses, or resources.
To offset this, you should take a more conservative approach and include contingency plans and buffer time to accommodate unanticipated changes.
Ignoring External Factors: Never ignore external factors such as regulation changes, technology, or economic shifts.
Otherwise, they utterly impact project results. Regular environmental scans and including these elements in your forecasting models are essential to guarantee a complete picture of possible risks and maybe opportunities.
Data Integrity Issues: Dependence on faulty or antiquated data seriously compromises accuracy. Maintaining data integrity through consistent validation and cleansing methods is essential. Use data management techniques to maintain high-quality data that accurately reflects the present project environment.
Static Forecasting Models: Don’t use static forecasting models that cannot change with fresh data. In dynamic project environments, static forecasts quickly become outdated. Instead, use constantly updated models, including real-time data, for more accurate and responsive forecasting.
Complexity Overload: Overcomplicating your forecasting model makes it difficult to maintain and understand, leading to mistakes and misinterpretation. Focus on simplicity and clarity, prioritizing critical variables that have the highest impact on your forecasts.
Read More: Top 7 Project Management Trends You’ll Regret to Miss In 2024
Types of Forecasting Methodology In Project Management
In project management, forecasting methodologies are categorized into two main types: Qualitative and Quantitative. Each approach serves distinct purposes and is best suited for different project environments.
Qualitative Techniques
Qualitative forecasting relies on expert judgment, market research, and scenario planning to predict outcomes, especially when historical data is sparse, or trends are not yet well-defined. This approach is valuable in fields like product development, where market conditions can change in a second and traditional data may not exist.
Expert Judgment
This technique taps into the knowledge and experience of industry professionals to make informed predictions. For example, in the early stages of developing new technology, experts might forecast potential adoption rates based on their understanding of market dynamics and consumer behavior.
While this method can provide valuable insights, it introduces subjectivity, leading to bias if not carefully managed. Therefore, it’s crucial to cross-reference expert opinions with available data and to be aware of potential cognitive biases.
Delphi Method
The Delphi Method is a structured communication technique that gathers input from a panel of experts over multiple rounds of questioning to reach a consensus. Each round allows experts to revise their answers based on the group’s feedback, gradually narrowing the range of responses.
This method is particularly useful in complex projects, such as the European Space Agency’s missions, where forecasting technological challenges and budgetary needs requires multiple expert perspectives.
However, one limitation is that the process can be time-consuming and may not always result in a true consensus if dominant opinions influence panelists or the rounds are insufficiently iterative.
Scenario Planning
This technique involves creating detailed narratives about future states based on different assumptions and variables. Scenario planning is especially useful in industries like energy or finance, where external factors such as regulatory changes or economic shifts can dramatically alter the course of a project.
Companies like Shell 3 have famously used scenario planning to prepare for various future oil market conditions, allowing them to remain resilient amidst market fluctuations.
The limitation of scenario planning is that it can be resource-intensive and might lead to over-preparation for scenarios that are less likely to occur, potentially diverting attention from more probable outcomes.
Best practices for qualitative forecasting include cross-referencing expert opinions with available data, regularly revisiting assumptions, and using a structured approach like the Delphi Method to mitigate bias. Real-world examples highlight the utility of these methods in projects where flexibility and adaptability are key.
Quantitative Techniques
Quantitative forecasting is data-driven and employs statistical tools to generate predictions. This is ideal for projects with a wealth of historical data and a need for precision, such as in construction, finance, or logistics.
Time Series Analysis
This method involves analyzing past data points to identify trends, cycles, and seasonal variations, which are then extrapolated to forecast future outcomes.
For example, in retail, time series analysis might predict future sales based on historical sales data, considering factors like seasonality and market trends. However, this technique relies heavily on the assumption that past patterns will continue, which may not always hold true in rapidly changing environments.
Regression Models
These statistical techniques model the relationship between a dependent variable and one or more independent variables. In project management, regression models can predict project costs or durations based on factors such as project size, team experience, or resource availability.
For instance, regression models might be used in the construction industry to forecast the cost of a new building by analyzing variables like square footage, materials used, and labor costs. The limitation of regression models is their dependency on the quality of input data and the assumption that the relationships between variables remain stable over time.
Monte Carlo Simulations
This technique uses random sampling and statistical modeling to estimate the probability of different project outcomes. It’s beneficial for risk assessment and decision-making in complex projects with many uncertainties.
In pharmaceutical development 4, Monte Carlo simulations are often used to forecast the success rates of clinical trials by modeling various potential scenarios. A key limitation is that Monte Carlo simulations require significant computational power and expertise in statistical modeling, which may not be readily available in all project teams.
Causal Models
These models predict outcomes based on the cause-and-effect relationships between variables. For example, a causal model might be used in an economic development project to forecast job creation based on investments in infrastructure.
These models are useful when the goal is to understand how changes in one variable might affect others, such as in policy analysis or marketing strategy development. The challenge with causal models is ensuring that the relationships between variables are accurately understood and that the model does not oversimplify complex interactions.
Read More: How to Reduce the Duration of Project Management
Key Components of Forecasting Methodology In Project Management
Accurate forecasting in project management hinges on three core components: cost, duration, and quality. Mastering these elements ensures projects are completed on time, within budget, and to the expected standards.
1. Project Cost Estimate
Cost forecasting is essential for preventing budget overruns. Bottom-up estimating is ideal for complex projects with well-defined tasks, as it breaks down costs to their most detailed level—an approach successfully used in the construction of the Empire State Building, where meticulous task breakdowns enabled precise budgeting.
For projects with accessible historical data, parametric estimating offers a quicker alternative by leveraging statistical relationships, such as cost per square foot in construction. This method is particularly effective in industries like aerospace, where historical cost data accurately informs new project estimates.
2. Project Duration
Accurately predicting timelines is vital for meeting deadlines. The Critical Path Method (CPM) is effective for projects with tight schedules, identifying the longest sequence of dependent tasks that must be completed on time to avoid delays.
For instance, CPM was instrumental in managing the complex assembly process of the Boeing 7775, ensuring key milestones were met. When dealing with uncertainty in task durations, the Program Evaluation and Review Technique (PERT)6 provides a probabilistic approach, offering a range of completion dates based on best, worst, and most likely scenarios.
This method was crucial in the Polaris missile project, allowing for flexibility in scheduling. These tools enable managers to create realistic schedules that account for potential delays and keep projects on track.
3. Project Quality
Quality forecasting involves setting clear standards and continuously monitoring them throughout the project lifecycle.
Quality Function Deployment (QFD) translates customer requirements into specific project deliverables. For example, in the development of the Toyota Prius, QFD ensured that expectations for fuel efficiency and design were met.
Failure Mode and Effects Analysis (FMEA) identifies potential quality issues early by analyzing possible failure points and their impacts—a technique commonly used in the automotive industry to design safety features.
Real-World Examples of Forecasting In Project Management
In project management, the effective use of forecasting methodologies can be the difference between success and failure. Let’s explore four insightful case studies that highlight the power—and potential pitfalls—of forecasting in real-world projects.
1. The Boston Big Dig Project
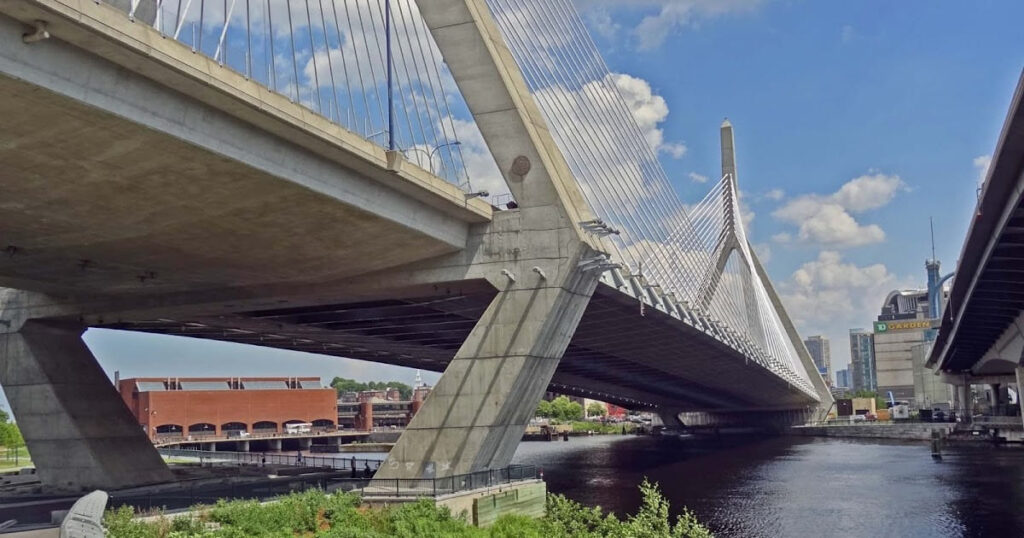
The Boston Big Dig 7 is a classic example of how inadequate forecasting can severely derail a project. Initially estimated to cost $2.6 billion in 1982, the project’s final cost escalated to $14.8 billion by the time it was completed in 2007.
The primary forecasting failures stemmed from underestimating the complexities of scope changes, inflation, and unexpected technical challenges. The lack of a robust forecasting system that could adapt to the evolving project complexities contributed significantly to these issues.
Tip: Implement a rolling forecast model that includes comprehensive risk management strategies, such as using Monte Carlo simulations, to account for uncertainties like inflation and scope creep.
2. CORE Business Technologies
CORE Business Technologies struggled with disconnected systems that hindered efficient forecasting and resource management. By adopting an integrated project management tool like Mavenlink, the company saw a 50% increase in productivity 8 and significantly improved its resource forecasting accuracy.
Mavenlink provided a centralized platform that streamlined data flow and improved the visibility of project metrics, which was critical for enhancing forecasting outcomes.
Tip: Evaluate your current project management tools for integration capabilities and data synchronization to ensure seamless forecasting and resource planning. Consider using platforms that offer real-time data insights and comprehensive project-tracking features.
Read More: Who Should Project Manager Interact With When Doing Integration?
3. Indianapolis Library Construction
During the construction of the Indianapolis Library9, inaccurate forecasting during the early foundation phase led to massive cost overruns and delays, with an additional $52 million in expenses and a two-year extension.
The failure to accurately forecast and manage risks early on severely impacted the project’s success. This case underscores the importance of integrating risk management with forecasting processes to anticipate and mitigate potential issues.
Tip: Conduct thorough risk assessments at the project’s outset and apply conservative estimates to account for worst-case scenarios. Employ forecasting tools that incorporate risk management features, such as PERT analysis or risk-adjusted project scheduling.
4. TeleTracking Technologies
TeleTracking Technologies faced challenges with outdated systems that hampered effective project forecasting. By transitioning to a more advanced project management platform, they increased their billable utilization by 37% 10 and improved overall project forecasting capabilities. The new system allowed for better data-driven decision-making, which was crucial for their success.
Tip: Regularly review and upgrade your project management systems to keep pace with technological advancements that can improve forecasting precision and overall project efficiency. Opt for systems with predictive analytics and resource management functionalities to enhance forecasting accuracy.
Conclusion
Effective project management isn’t just about reacting to challenges—it’s about predicting and preparing for them. Accurate forecasts let you anticipate difficulties, allocate resources efficiently, and keep budgets and schedules on track.
By blending qualitative and quantitative forecasting methods, you can minimize risks, build stakeholder trust, and dramatically improve your project success rates. It’s about turning uncertainty into actionable insights that ensure your projects are delivered on time, within budget, and to the highest quality standards.
Curious about what’s next for project management? Check out our insights on the 8 Project Management Trends to expect in 2025 and stay ahead of the curve!
References
- Dwivedi, R., & Dwivedi, P. (2021). Role of Stakeholders in Project Success: Theoretical Background and Approach. Deleted Journal, 11(Issue 1), 38–49. https://doi.org/10.35808/ijfirm/248 ↩︎
- Langley, M. (2017). Transforming the high cost of low performance. In 9th Global Project Management Survey. Retrieved August 12, 2024, from https://www.pmi.org/-/media/pmi/documents/public/pdf/learning/thought-leadership/pulse/pulse-of-the-profession-2017.pdf ↩︎
- Cabanes, B. (2023, November 6). Case study: how Shell anticipated the 1973 oil crisis. Polytechnique Insights. https://www.polytechnique-insights.com/en/columns/society/case-study-how-shell-anticipated-the-1973-oil-crisis/ ↩︎
- Yu, M. J. (2012). Simulating the drug discovery pipeline: a Monte Carlo approach. Journal of Cheminformatics, 4(1). https://doi.org/10.1186/1758-2946-4-32 ↩︎
- Alkhaili, M., Gul, R., Khalaf, R., & Iqbal, S. (n.d.). The Boeing 777 Program: A Project Management Feat. PDXScholar. https://pdxscholar.library.pdx.edu/etm_studentprojects/2117/ ↩︎
- Saha, S. (2023, December 11). [Solved] Boeing 7E7 Case Study Solution: WACC Calculations and Answers to 7 Questions – SimpliMBA. SimpliMBA. https://www.simplimba.com/boeing-7e7-case-study-solution/ ↩︎
- Haynes, W. (2008). Boston’s Big Dig Project: A Cautionary Tale. In Bridgewater Review (pp. 3–7). https://vc.bridgew.edu/br_rev/vol27/iss1/5 ↩︎
- CORE Business Technologies Increases Billable Utilization by 35% with Mavenlink – Mavenlink Marketing. (n.d.). https://www.mavenlink.com/blog/article/core-business-technologies-increases-billable-utilization-by-35-with-mavenlink ↩︎
- Adam, Abderisak & Josephson, Per-Erik & Lindahl, Goran. (2015). Implications of Cost Overruns and Time Delays on Major Public Construction Projects. 10.1007/978-3-662-46994-1_61. https://www.researchgate.net/publication/300629545_Implications_of_Cost_Overruns_and_Time_Delays_on_Major_Public_Construction_Projects ↩︎
- Case Study: Carilion Clinic – Teletracking. (2024, April 17). Teletracking. https://www.teletracking.com/resources/case-study-carilion-clinic/ ↩︎